Commodity trading analytics: exploiting a new set of options
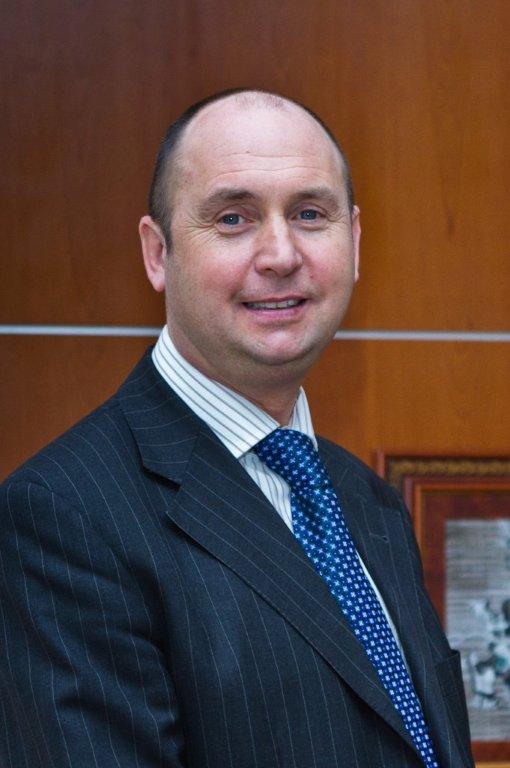
Edwin Van der Ouderaa, managing director, analytics for financial services, Accenture EALA.
Every trader tries to factor in the range of possibilities that can affect their market position, but commodity traders have it tougher than most. From lingering uncertainty over how the Middle East situation affects oil prices, through to how floods, droughts, crop reports, pirates and other factors feed into the prices of key commodities from cotton to corn. Added to this, constantly shifting costs of shipping, an endless stream of social media posts, and 24×7 markets all serve to heighten volatility and the scope of the challenge, writes Edwin Van der Ouderaa.
Unsurprisingly, then, commodity traders are rapidly adopting new analytics tools to help, testing how a combination of powerful cloud-based processing systems and increasingly sophisticated analytics tools can boost their bottom line. On the surface of it, there is clear potential: from optimising asset valuations, right through to more accurately foretelling the commodity price movements.
But while many commodity traders are focused on the expanding capabilities of these tools and systems, there is a different challenge to getting this right: working out the right questions to ask.
This requires a deliberate approach to the pre-analysis stage, not least by taking into account the firm’s strategic business goals. For example, if a trading organisation is part of a public company, understanding how performance is driving shareholder value would be a clear important strategic objective that needs to be assessed.
Bearing this in mind, management can seek to explore a range of new questions, which were previously just not feasible to assess—or too complex to calculate quickly enough. For example:
- What is the estimated impact of the trading portfolio on earnings per share? How effectively have positions hedged forecasted asset output?
- How does the return on equity or return on invested capital within the trading business compare with internal targets? How does it compare with peers?
- What level of catastrophic risk exists within the trading portfolio and how may that impact current and future earnings? How much of this risk can be insured using market instruments and what can’t be? How much of these risks are attributable to credit versus price versus operational risk? How are these risks interdependent?
Gaining insight into these kinds of questions can be significant, not least given how quickly sizeable trading profits, or losses, can tally up. In 2005, for example, one hedge fund bet that natural gas prices would rise following Hurricane Katrina, but quickly racked up $6.5 billion in losses when they didn’t. At the same time, a separate fund just as quickly generated $1 billion profits betting the opposite way.
As these examples suggest, any new insights can prove to be highly material for a trading business—and not just in terms of risk exposure. Take the issue of comprehensive cost management, as one example. As traders strive to improve profitability in volatile times, data analytics can pinpoint cost reduction opportunities around the transportation of commodities, inventory, tariffs and financing—from shifting warehousing costs in one part of the world, through to a gap to exploit in the price of shipping elsewhere. By using cost-related analytics to determine if spending is commensurate with business goals, firms can better optimize the use of resources, driving more efficient value creation. Similarly, by utilizing cash forecasting tools and working capital records, trading companies can minimize their cost of capital.
Of course, while analytics tools and services can be deployed to answer a wide range of questions, they have to be focused on specific goals. Just as a scientist must set out a hypothesis prior to setting up complex experiments to test it, so too must analytics users. Many service providers claim to have packaged solutions that take the guesswork out of this process, but these tools rarely provide the insights or value that firms are looking to achieve. Quite simply: in the diverse world of commodity trading, where organizational types, sizes and strategies can vary so widely, one size does not fit all – tailoring counts.
While most analytical tools have advanced simulation and mathematical engines that can answer a wide variety of queries, each tool must be configured properly upfront to derive a useable answer. Despite the vast computing power available via the cloud, some simulations can still take hours to perform if the right data inputs are not in place, or if a company’s data warehouse is not calibrated properly. All of this matters, not least in an environment used to working in split seconds.
Furthermore, trading firms still need to invest the time in getting the right data. For example, even for a trader simply looking to understand how their current trading strategy might impact the firm’s EPS, they’d likely need some or all of the following data: current trading positions; current and historical settlement and forward pricing; trading volumes; mark-to-market valuations; interest rates; shares outstanding; forward price curves; and on and on.
Despite all these challenges, the emergence of new analytical technologies is allowing trading organizations of all sizes to unearth trends that can improve future profitability. The most important factor in unlocking the value of such analytics, though, doesn’t lie in the choice of tools, but rather in clearly defining the opportunities and issues that the firm is trying to address. Getting this right may well become a key differentiator between success and failure in a firm’s commodities strategy.