Piecing together the data scientist puzzle
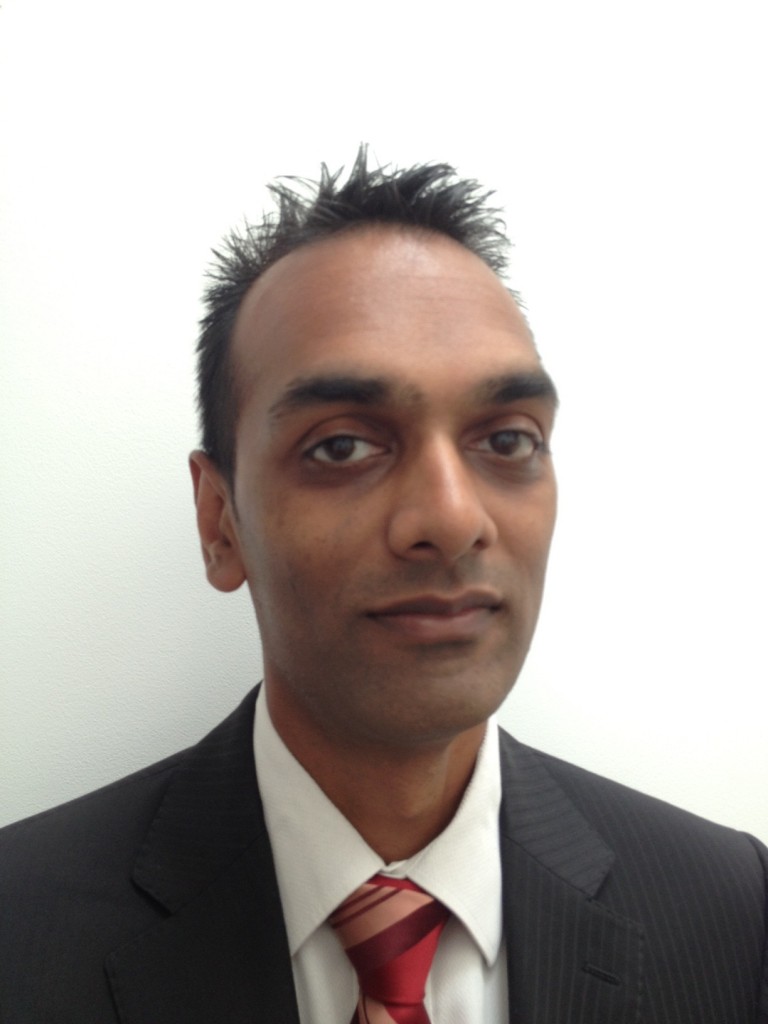
Dev Bhudia is vice president of product management at GoldenSource
Depending on who you speak to, the definition of a data scientist seems to mean different things to different people. Some see it as a glorified number crunching role, others believe the position requires someone more inquisitive to spot and respond to key trends. The role can also be linked closely to the chief data officer, but that position is more about devising an enterprise-wide strategy to share the best data across the business, writes Dev Bhudia.
The truth is that when people refer to the role of a data scientist, they essentially mean people who examine the interrelationships between diverse sets of data as well as the disparate systems, processes and locations which house them. Across certain sectors, such as retail, the role is very mature. For some time now, this has been a space that has mastered the art of using the right information at the right time. Amazon is the blueprint for this: by analysing behaviour across multiple accounts, it knows exactly when and why to push a certain product to a customer. However, it is a slightly different story in financial services, where the role is a little bit like an unformed puzzle: all the pieces are there, it just hasn’t been put together.
One of the reasons for this unformed jigsaw is the inherent complexity of the industry, with so many different areas needing the position to fulfil specific tasks. Take algorithmic trading. To date, this is the one area which has seen the data scientist excel. This is where data scientists, or quants as they are more commonly referred to, look for trends to build highly complex computer models to beat human traders on the markets. Without data scientists, this form of market making, responsible for so much liquidity across global markets, wouldn’t be possible. But while the front end trading desk is reaping the rewards, the sector as a whole, including the middle and back office, has only scratched the surface when it comes to deriving value from the vast quantities of information at their disposal.
In order to link the pieces across the entire financial services space, there has to be someone within an institution that focuses on looking for the relationships between data across disparate sources. Did the price spike due to a corporate action, or did it fall because a rating dropped for an issuer? What is the effect of this drop to the bottom line? While all the required skillsets already exist across the sector to find the answers, the first step is to find a way to piece them all together.
Financial institutions have people with the skills to do modelling and statistical analysis, but this needs to be married with the skillset of someone who is able to spot key trends. At present, the two pieces aren’t coming together. Once they do, the final piece is ensuring that they have the right tools to mine through the different data sets. It is no good having the combined skills if the technology isn’t underpinning them. Only once the puzzle is fully formed, will we start to see the rise of the data scientist in across the wider financial sector, similar to what we have witnessed in other industries.