Sibos 2018: AI – efficiency is just clever laziness
Sibos kicked off the first day hosting a panel about artificial intelligence (AI), which naturally was filled with jokes about Skynet and the robot uprising. Speakers just can’t resist themselves, can they? Despite this, the three panellists reiterated the impossibility of a sentient, free thinking AI with the current computing power in the world.
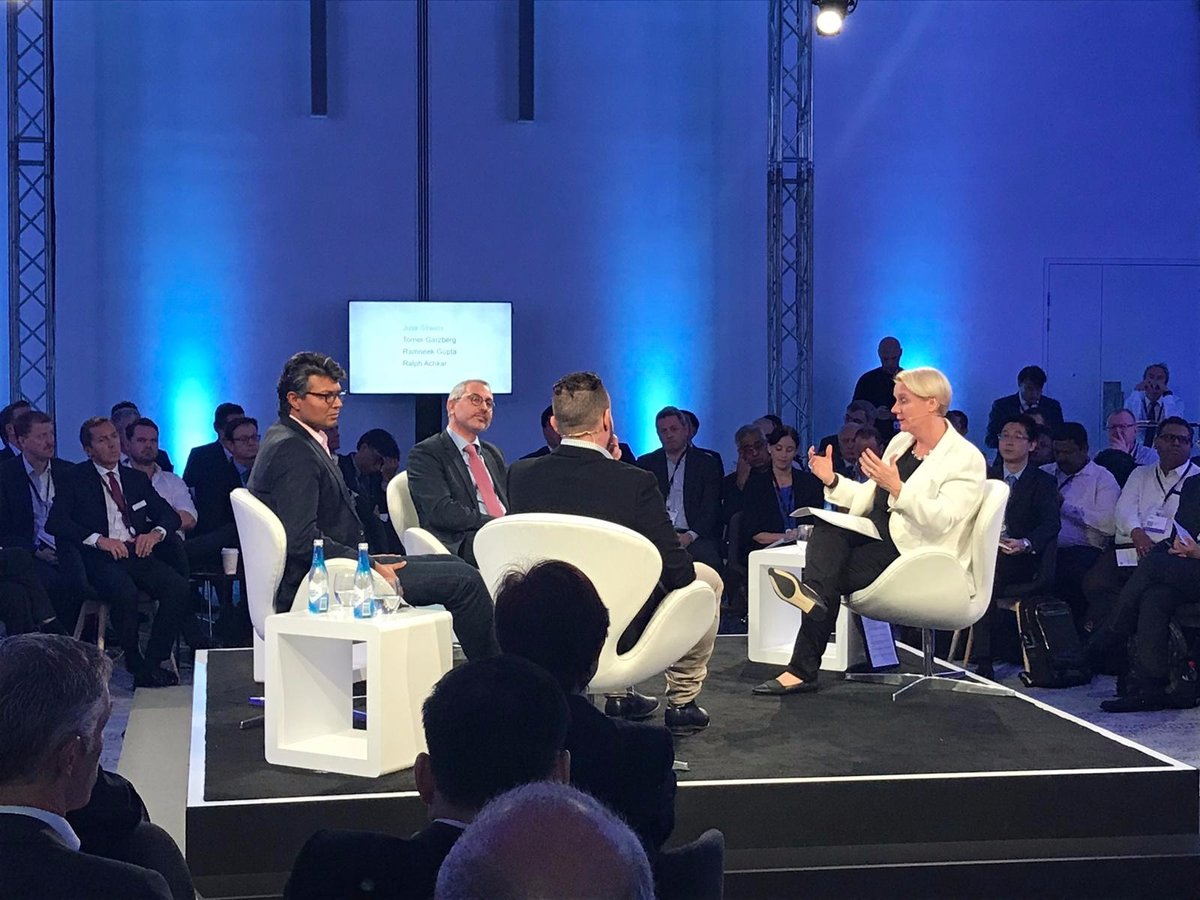
AI and the economy of labour
However, the main point of this panel was slightly different to what we see in every other talk about AI: the economy of labour. Tomer Garzberg, CEO and founder at Gronade, said that the way we work is broken.
He just seems to be one of the many who believe that increasing operational efficiencies is the most achievable milestone, as proved in the audience survey that the panel held right there and then. Increasing the business pipeline in new markets, as well as enhancing insights and the pipeline in current ones, were relegated to a very small minority of the audience’s prospects.
In the coming years, AI will displace 75 million jobs. This may sound like a tragedy, but on the flipside, it will also create 135 million new ones – one of the little facts that go unnoticed around the topic.
But from a more practical perspective, AI can help us rethink what we dedicate our time towards. At an enterprise level, lots of time and resources are invested into areas that can be simplified by the magic of automation. Where to start in the quest for automation? Garzberg said that the low-hanging fruits are always the safest investments, where businesses “bleed” the most.
These usually come in the form labour efficiency and cost of service. But the number of applications for AI is increasing by the minute. Garzberg talked about the very plausible scenario where tech can spot the customers that are being underserved and are most likely to start leaving your business and address it “before they even know they want to leave”.
Ramneek Gupta, MD at Citi Ventures, pointed out that machine learning (ML) and pure AI work at diametrical points of a spectrum, where ML, unlike AI, requires a lot of computing power, but is not able to create a conceptual model of the world from which it can learn from.
The latter, however, requires a lot of practical and contextual data. Ralph Achkar, MD at State Street, explained that clearing, cleaning and processing data will be key if we want AI to keep developing to newer more intricate forms of cognitive computer processing. “It will be the new blue-collar jobs,” highlighted Garzberg.
This article is also featured in Daily News at Sibos 2018, our flagship daily publication at the Sibos conference.
Click here to see the Daily News at Sibos editions – they are all free to read!
Follow us on Twitter @DailyNewsSibos