Teradata Analytics Universe 2018: deep learning and card tricks
US-based Teradata believes that the time for deep learning (DL) is now thanks to algorithmic breakthroughs.
On day three (16 October) of the Teradata Analytics Universe 2018 (14-18 October) event in Las Vegas, FinTech Futures soaked up the sessions and gained some insights on artificial intelligence (AI) and DL.
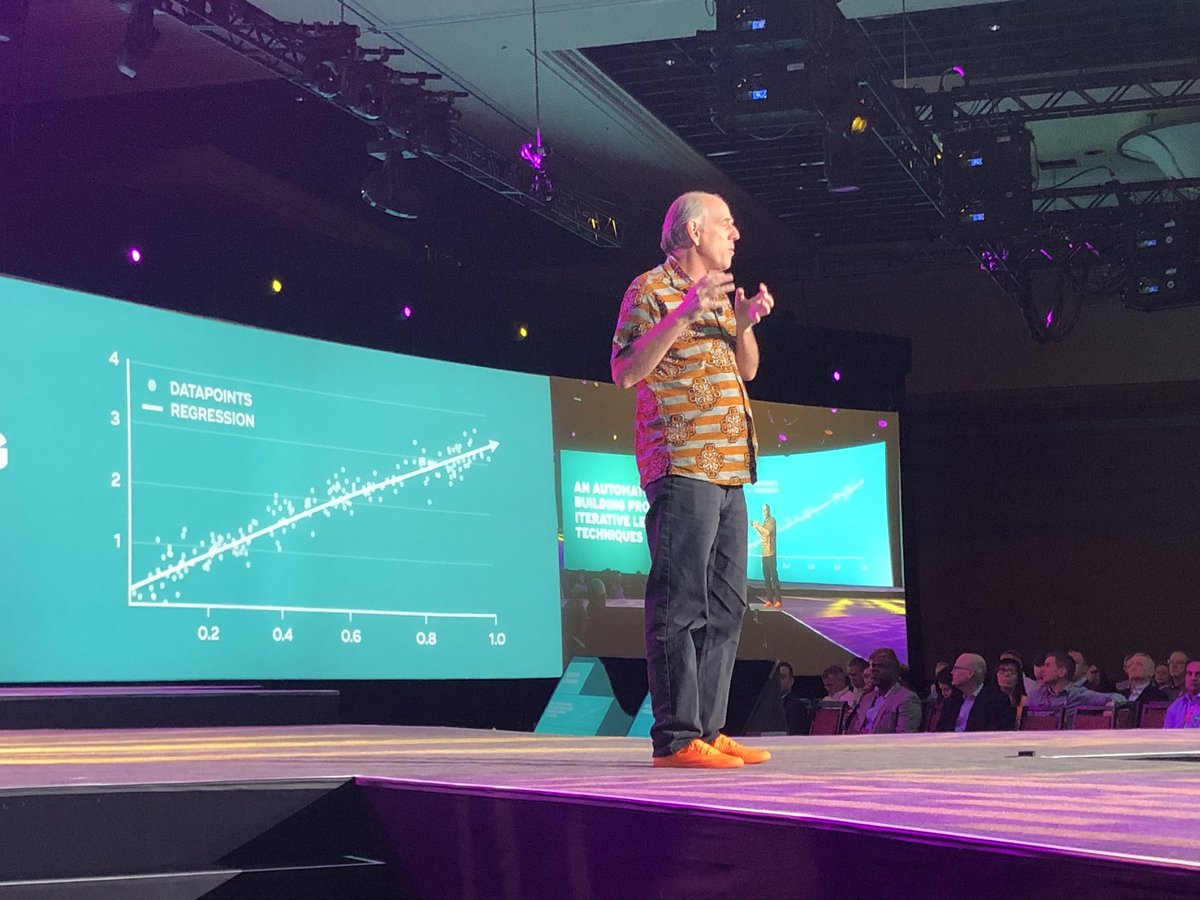
Stephen Brobst, Teradata’s CTO
In our first report, the company unveiled a new logo and theme – “rise above” – as it sought to be the solution to the complexity of analytics.
The second report outlined the firm’s ambitions in the fintech space – such as a mysterious US bank deal and conversations with potential clients.
The event at the Mandalay Bay Hotel is impressive – over 3,000 attendees from 50 countries; and some good walking exercise due to the size of the convention centre.
DL, ML, PI
In the keynotes at the general session, Stephen Brobst, Teradata’s CTO, offered up pragmatism to the large gathering when it comes to DL.
Here we can give the firm some credit as last year it was a similar message. Teradata’s staff are keen to show off their knowledge (and it is formidable at times), but they don’t pretend that everything is going to be easy or simple cures are out there.
In Brobst’s view, 95% of machine learning (ML) work is the same that has been done for decades. The maths is largely the same – but the “magic” is automating the process and bringing in self learning.
He calls AI the “most overhyped” term in the industry and wants people to be realistic. Brobst reckons DL is different from traditional ML as the latter involves linear mathematics – the type “my nephew knows how to do”.
But with DL there is a “transformation of data” – comprising hidden and neural layers, which brings in non-linear data. This creates a more robust model. However, he reminded the crowd that DL is hungry for lots of data so “set expectations appropriately”.
Brobst, dressed like Tom Selleck from the 80s TV show ‘Magnum PI’, didn’t keep his thoughts private.
He asked – why DL now? Well, the “AI winter is over when funding dried up – but recent breakthroughs on the algorithm side mean binary neural networks can be mapped to GPUs”.
This means a highly parallel system – “very stupid, very cheap” – and people can scale DL for real applications.
As an example, he turned to “boring” banks and the matter of credit card transactions. Think of the date stamp or merchant category involved in this.
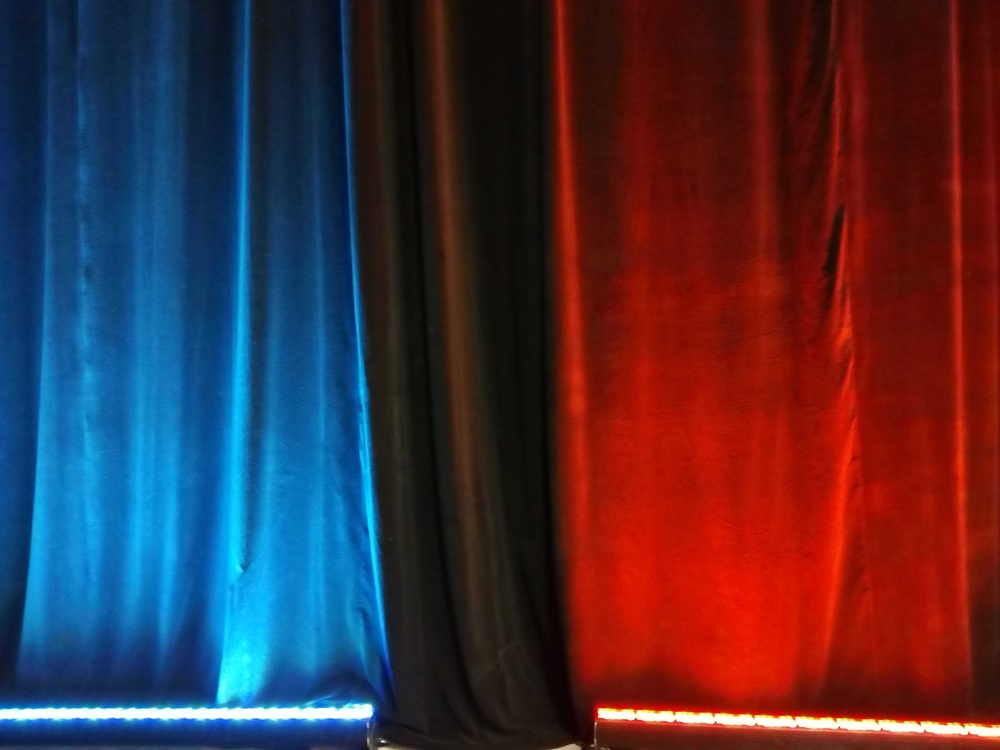
Love the ‘Twin Peaks’ look
With image recognition for bank transactions, it can apply some simple maths and map pixels to an image. He showed us an image of non-fraud and fraud that was clearly different even to the human eye.
As with others at the event, he cited last year’s Danske Bank deal. The bank uses an AI-driven fraud detection platform and has upped its detection rates. I hear about this bank a lot, so it might be time for Teradata to find another bank as an example to show off more of its clients.
In fact, in a separate session at one of the ‘Twin Peaks’-styled innovation theatres, Ben MacKenzie, director AI engineering at Teradata Consulting, discussed applying DL to credit card fraud.
MacKenzie believes that when it comes to NLP and image recognition, DL is doing very well. Some advantages include less features for engineering and better generalisation.
So while people are good at image recognition, they are not when it comes to looking at tabular data. That would be quite a boring task for the human mind, yet DL can take it on.
Contradictions
If you’re keen on AI, then stick around as there is more to share.
In another session on “What does it take to make AI work for you”, Dan Vesset, group vice-president of IDC’s Analytics and Information Management practice, felt that the technology is “pervasive” yet also “nascent”. Ultimately, the tech world is rife with contradictory statements at most conferences.
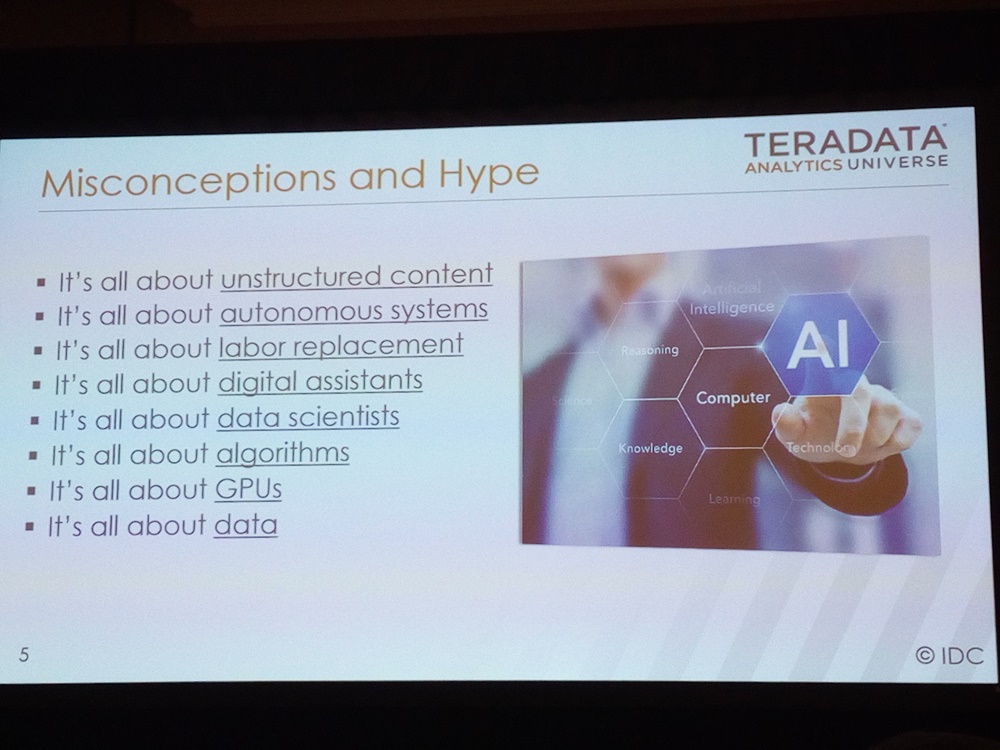
It’s all about AI
Based on IDC research, AI penetration is still low. Only 21% of enterprises (1000+ employees) have developed conversational AI tech. In addition, when it comes to use case spending on AI in 2018, automated customer service agents came in top at $2.3 billion. Fraud analysis and investigation was $1.12 billion.
Realism reared its head again, as Vesset says expectations of perfection need to be dampened. For example, face ID technology has been beaten by a mask.
A common theme is the lack of data scientists (DS), in IDC research from 2017, 61% of organisations surveyed did not have a DS in their organisation. Some firms offer the tech to automate the DS role, such as Google and DataRobot.
None of the presenters have been replaced by automatons or robots… yet.
It was another good day for sessions but I have pressed Teradata to get interviews with the COO or CTO to discuss fintech in more detail. Call it deep fintech learning if you like… and I am a willing student.
There will be more reports from the Las Vegas show as FinTech Futures is here all week.