Separating the wheat from the chaff
SPONSORED INDUSTRY INSIGHTS
Leveraging high-value content from vast unstructured data lakes presents one of the biggest barriers to operational efficiency. Peter Duff (CEO, Adlib Software) looks at the pitfalls of dealing with complex data estates and where the road to successful digital transformation lies.
In chaos theory, initial conditions have a critical bearing on outcomes. Dealing with unstructured content is no different. If an organisation’s data estate is in good health – and AI, RPA and analytical tools can efficiently mine content – you see better results radiate outwards.
On the outer periphery, that means a more coherent customer journey, accurate insights, and increased customer response times and overall satisfaction. Operational efficiency tightens up and cost savings can be achieved. This is particularly relevant for organisations sitting on eons of complex legacy customer data, such as we see in the banking and insurance sectors.
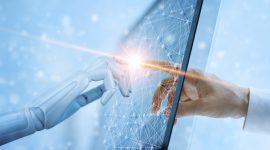
Harnessing IBM Watson for risk analysis is one powerful tool at your fingertips.
Firstly, let’s look at analytics: when they’re rapid and agile, businesses have better oversight and a clearer vision ahead. If your data lake is an inaccessible mess, even the best analytical tools won’t perform well.
Robotic Process Automation (RPA) is another major consideration. Without being able to access and leverage high-quality data quickly, businesses can’t make the most of all RPA has to offer. Ultimately, this means leaving the door open to inefficiency and risk.
An example, for a bank, may be as follows: the banking institution wants to automate their customer onboarding process to improve operational efficiencies by leveraging RPA. The goal is to deliver an enhanced digital experience that accelerates response times and improves overall satisfaction. But if RPA can’t extract accurate information from a mass library of unstructured data, the firm won’t make much headway with their objectives.
The same can be said for AI: a chatbot relying on high-quality customer data may compromise operational efficiency and customer expectations if it can’t access the right content. Sub-par chatbot performance means customer inquiries get diverted to key resources. Here, you’re not only disrupting and prolonging the customer journey, but you’re also diverting a knowledge worker away from high-impact activities. Put simply, the cognitive capability of customer-facing AI might be there, but without access to quality content, it can’t do its job.
Coming back to insurance and the utilisation of AI in a non-customer-facing scenario, imagine you’re a re-insurer harnessing IBM Watson for risk analysis and predictability; that’s one powerful tool at your fingertips. Yet no matter how exceptional the cognitive capabilities of Watson are, if data – both structured and unstructured – can’t be leveraged efficiently, Watson can’t run at peak performance. Not only does this mean you’re not seeing a good return on AI investment, you might be compromising your ability to effectively model products and pricing.
In summary, unstructured content sitting in swelled and complex data lakes presents major challenges for RPA, AI and other tools. Distilling this information – separating the wheat from the chaff – is at the centre of operational efficiency across the board. Particularly for the financial services sector, how data is managed, cleaned and accessed at source has a massive radial effect on operational efficiency and bottom-line.
This is also where unstructured content experts such as Adlib Software come in. They enable businesses to create an end-to-end digital strategy by discovering, accessing and transforming unstructured data into high-quality structured data that fuels process automation and business intelligence.
By Georgina Treloar, freelance writer and editorial contributor to FinTech Futures.
This article is also featured in the summer July/August 2019 issue of the Banking Technology magazine.
Click here or on the banner below to read the digital edition – it is free!