Why has mid-market lending stagnated in banking?
If you look at the global small and medium-sized business lending market today, a similar pattern emerges: when it comes to loans of $500,000 or less, big banks and platforms such as Funding Circle, Kabbage, Ant Financial, Lending Club, Iwoca, etc. offer several debt options including small general-purpose business loans, asset finance, and invoice finance.
To make this commercially viable, lending is typically based on automated credit models which allow lenders to process loans quickly and efficiently.
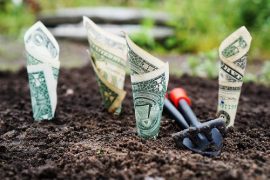
SME lending has been overlooked and underserved.
At the larger end of the market (loans of $25m+), large banks can justify allocating significant amounts of time and resources to underwriting the loan because the potential returns are substantial. However, borrowers are typically required to offer property as collateral – an approach that simply isn’t fit for purpose in this age of falling home-ownership and new industries such as tech where property assets aren’t required. Banks tend to be unwilling to accept other assets (IP, stock, debtors, plant and machinery, etc.) for loans of this size, as the potential returns don’t justify the complexity of underwriting needed.
Loans that fall outside these parameters (i.e. those between $500k and $25m), are either too large to be subject to the automated credit process that can be undertaken with smaller loans (as it is difficult from a risk perspective to justify automating this size of loan); or too small to be underwritten in the way that big banks do with large loans because the potential returns don’t make it commercially viable. As a result, small and medium-sized businesses have been overlooked and underserved for decades.
One potential fix for this issue is the use of machine learning fintech. By collecting millions of available data items on SMEs across various parameters, sectors, and markets, and banks can use algorithms to identify data that lenders need to make more informed credit decisions through detailed line item underwriting.
While the industry may never achieve 100 percent automation of complex credit analysis using AI and machine learning, doing so may not be a worthy goal. Rather, there are numerous advantages to be gained from the added insight and efficiency that these techniques can bring to the lender and the borrower in the process.
The benefits to the lender from the adoption of big data, AI and machine learning technologies primarily stem from what they enable them to do as a lender. Firstly, because they can analyse more complex credit situations, they are set free from the shackles of their rigid credit product suite. They should therefore be able to gain market share by having a differentiated product offering. Secondly, enhanced analysis and better use of external data should lead to better credit outcomes from a more objective, data-driven credit assessment process. Thirdly, they should see greater efficiency from a streamlined, automated process where computers do what they do well (process large amounts of data) and humans do what they do well (provide judgement and expertise).
Used wisely, these technologies can help to remove the friction that has prevented this from happening in the past. Finally, since they are no longer reacting to the market and fighting to establish a niche in a very competitive space, and can act at speed, they should see margins improve.
Lending practices in financial services are in a rather unique position in the market, as some audiences of lenders have seen countless innovative new solutions in the market while others are bogged down in decades-old credit practices. There is untapped potential in mid-market lending that banks need to seize upon using, and machine learning platforms will be the mechanism with which they do.
By Sean Hunter – CIO of OakNorth