AI: Understanding bias and opportunities in financial services
It is undeniable that our lives have been made better by artificial intelligence (AI). AI technology allow us to get almost anything, anytime, anywhere in the world at the click of a button; prevent disease epidemics and keep them from spiralling out of control, and generally just make day-to-day life a bit easier by helping us to save energy, book a babysitter, manage our cash and our health all at a very low cost.
AI’s penetration into systems and processes in virtually all sectors of business and life has been rapid and global. The speed and scale at which AI is proliferating does however raise the question of how at-risk we may be that the AI we are building for good can also be introducing damaging bias at scale.
In this two-part series, I explore the issues with AI constructs, the good bad and the ugly and how we can think about shaping a future through AI in financial services that helps lift people up rather than scaling problems up.
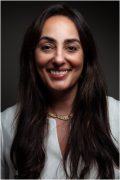
By Nadia Sood, CreditEnable
Part One
AI in financial services: The good
From using predictive analysis to forecast consumer spending and advising on personal wealth management, to underwriting loans and transaction monitoring – AI’s footprint in financial services can be seen everywhere.
AI that has focused on better understanding of customer’s needs and security can have substantial benefits for consumers and several banks have already introduced innovation in this space.
In 2018, Goldman Sachs acquired a personal finance app called ClarityMoney. The app pulls users’ transaction information to remind them of spending goals, flags transactions that it finds unusual for a given account, and also moves money into savings for users. It also calculates how much users could save if they cancel some recurring fees in their bank account and even allows users to cancel unwanted subscriptions in just a few steps. This kind of technology revolutionises tracking personal finance.
NetOwl is a suite of entity analytics products used by Royal Bank of Canada (RBC). It analyses big data in the form of reports, social media, as well as structured entity data about organisations and places. It uses tools such as semantic search and discovery, compliance monitoring, cyber threat monitoring and risk management. It can even translate names written in foreign languages, perform name matching, and identity resolution. RBC uses the company’s tool EntityMatcher as part of its fraud detection and prevention efforts. Using this software, RBC is able to screen potential new customers against a large set of individuals who have perpetrated fraud against such organisations in the past. NetOwl is able to quickly and accurately match newly identified perpetrators against millions of records. This kind of technology not only benefits the bank using it, but also helps reduce the likelihood that nefarious organisations penetrate the institutions that the rest of society needs in order to function.
What can go wrong
While this technology offers immense benefits, it can equally help perpetuate unhealthy biases. Imagine that your expense tracking software was used by your bank to determine whether you should be eligible for a loan product, but filtered out all people over the age of 50 because the algorithm was constructed by a young technologist assigning a value in an algorithm and who just assumed over 50s didn’t need loans? This would not bring a benefit to over 50-year olds or to the banks who would be missing out on a huge part of a creditworthy pool of customers.
Real world examples of this type of bias creeping in with detrimental consequences to women and minorities have already occurred and at scale.
In 2014, Amazon developed an internal AI tool for selecting the most promising candidates by examining their job applications, particularly their CVs. However, the software quickly taught itself to prefer male candidates over female ones, penalising CVs that included the words ‘women’, which would often refer to women-only clubs. The software also downgraded graduates from two all-women colleges. This issue stemmed from the fact that this software was trained on data submitted by mostly men over a ten-year period. Despite attempts to fix the bias, Amazon eventually lost faith in the impartiality of the system and abandoned the project. Commenting on this issue, John Jersin, VP of LinkedIn Talent Solutions stated that AI is not ready to make a hiring decision on its own – the technology is not ready just yet. The real issue with the AI that was deployed wasn’t that it wasn’t ready, but rather that the starting point was flawed. The data set used should have included an equal set of data on women and on men, and because it didn’t, the eventual decisioning tool that was constructed by Amazon ended up with an inherent bias against women.
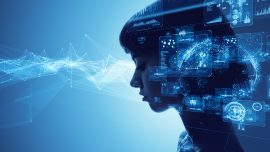
These systems are often tested predominately on white men.
A similar issue has occurred in the area of AI for facial analysis. A computer scientist and MIT graduate, Joy Buolamwini, found that facial analysis software from tech giants such as IBM, Microsoft, and Amazon, could not detect her dark skin. Her face was only detected when she put on a white mask. This is not surprising as these systems are often tested predominately on white men.
After testing facial recognition from these tech giants on various faces, Buolamwini found that all companies performed substantially better on male faces than female faces – and darker-skinned female faces did substantially worse. For lighter skinned men, she found an error rate of less than 1%. However, this figure rose to 35% for darker skinned women. These AI systems also failed to correctly classify the faces of Oprah Winfrey, Michelle Obama, and Serena Williams despite the fact that these women are some of the most famous people in the world and generate some of the most significant number of images on the internet.
In both these examples, the institutions building the AI could have been smarter about the data-sets they used to form their conclusions and train their AI and better about including more diversity in the groups of people who were building the systems.
AI in banking is not immune to this risk. The trick is going to be how to develop AI that doesn’t perpetuate widespread bias that exists today especially in the area of gender.
Gender bias in banking services is clearly seen around the globe. A European study found that businesswomen are less able to access loans from banks than businessmen. Male entrepreneurs in Europe are 5% more likely to successfully get a loan for their business from banks than women. Even those women that are able to access loans are subjected to higher interest rates, with an average of 0.5% more on a business loan than men. It is not the case here that women are worse at business than men and so present worse credit risks – the average venture-backed technology company run by a woman is started with a third less capital yet yields annual revenues that are 12% higher than those run by men.
The substantial social benefit of AI if applied properly is that it can help spotlight the strong performing, good eggs in the lending basket. For instance, it can read between the lines in deciding whether to lend to an individual earlier excluded by a lending officer because the entrepreneur is a woman, especially since the gender of the entrepreneur has nothing to do with the ability of the individual to repay debt. AI can help eliminate the discrimination arising from cases such as this.
At a societal level AI stands the chance of democratising the access to capital for women and minorities. But AI needs to be developed in a consequent thoughtful manner for this promise to be delivered on.
In the second part of this series, I explore how AI can be applied as a force for good by financial institution to expand the pool of clients to be more inclusive.
An objective analysis can highlight what gender biases may cloud – and banks would thus be less likely to filter out women-owned businesses without first being made aware of their merits and creditworthiness. With time, AI can be a transformative tool in shrinking these biases.
By Nadia Sood, CEO, CreditEnable. In this two-part series, Sood explore the issues with AI constructs, the good bad and the ugly and how we can think about shaping a future through AI in financial services that helps lift people up rather than scaling problems up.
This article is also featured in the October 2019 issue of the Banking Technology magazine.
Click here or on the banner below to read the digital edition – it is free!