From data to decisions: The rise of AI in retail banking
Artificial intelligence, or AI, has undoubtedly been the 2019 buzzword in financial services. While not new – the term “artificial intelligence” was coined in 1956 – AI is seemingly becoming the future of everything.
The hype around its potential is certainly justified economically. According to a recent report by Autonomous Next, the cost savings achieved through AI applications across financial services – including banking, investment management and insurance – are expected to reach $1 trillion by 2023; $447 billion of which would be realised in the banking sector alone.
Currently, one of the aspects of AI most applicable to banking is machine learning. Broadly speaking, machine learning enables systems to automatically learn from the huge quantities of customer data it collects and translate that knowledge into algorithms. With financial services providers having to manage an increasing volume of data points from multiple sources, cutting-edge data analytics and processing capabilities are increasingly becoming crucial differentiators.
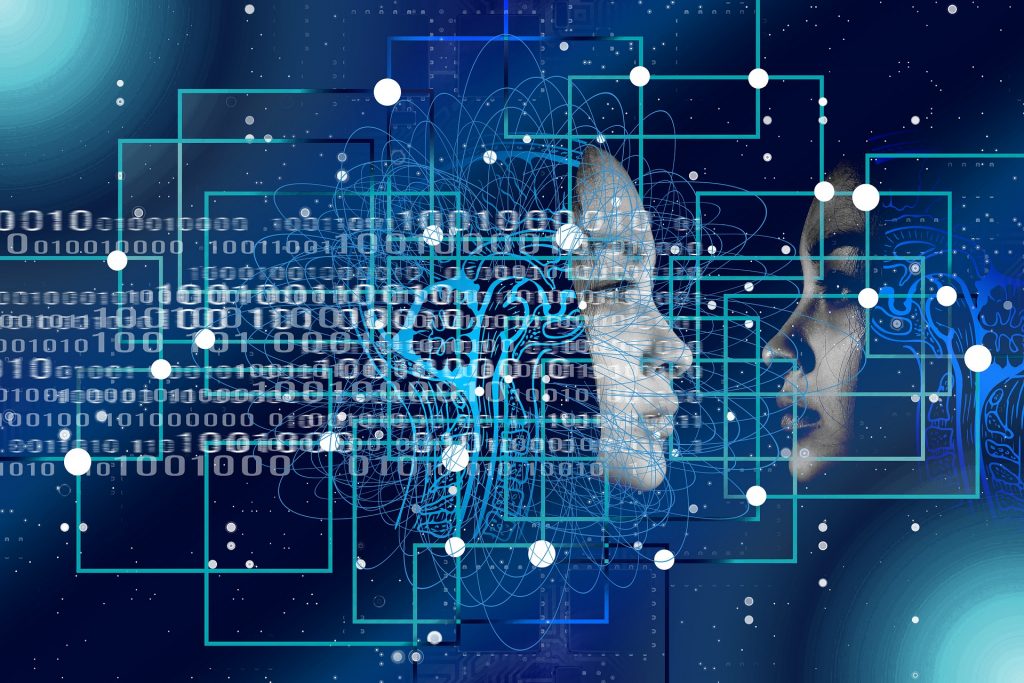
But first, banks need to de-silo their data and create a single data universe.
But first, banks need to de-silo their data and create a single data universe. CaixaBank consolidated its 17 data marts into one, which has more than 900 terabytes of information and can handle over 12,000 transactions per second during peak hours, enabling the advanced analytics and business intelligence required to extract value from the data and providing the agility required to drive future digitalisation.
In the short term, AI-tools can help simplify and personalise client services and thus enhance customer experience. A subset of machine learning technology, natural language processing (NLP), has already become ubiquitous among banking services providers in this regard. NLP powers robo-assistants and chatbots that are widely used among global retail banks. These tools can interact either through text or voice and rely upon advanced NLP systems to turn the complex human verbal expressions into machine-readable data, which allows the computer to promptly answer queries in a language that humans understand.
Not only does this offer an additional communication channel to customers, but when combined with machine learning systems these digital assistants are able to generate real-time recommendations tailored to individual customer needs. What’s more, with the application of another specialised machine learning subset known as reinforcement learning, based on the continuous learning of the machine itself, banks can “hyper-personalise” the offer to clients using a precise understanding of their preferences concerning the best moment (even time of day) to contact them, in the most suitable format, and on the most convenient channel. In the long term, the use of such tools can help banks revisit and rationalise their product strategies, focusing on profit generating activities, while ensuring continued client satisfaction.
Machine learning has also found practical applicability in the banks’ day-to-day client servicing processes such as the automation of payment processing.As an example, CaixaBank developed a machine learning-based system to manage its over €450 million annual direct debit payments. Previously, when a bill was sent to an account with insufficient funds, a human advisor would analyse the case and decide whether to allow, reject, or postpone the debit.
Now, the bank is using an AI system to make these decisions, significantly reducing the number of human hours required to manage client risk, allowing banking advisers to focus on value-add functions. The model currently operates in 600 branches and resolves incidents with 99% accuracy, meaning that the system virtually always thinks and acts like a real person. If the model were to be expanded to the entire CaixaBank network, it would save approximately 82,000 hours of manual processing work.
Another key area of AI applicability is the potential for AI to enhance the efficiency of service distribution networks within banks. Branch-based payments and cheque deposits have been steadily decreasing in the developed world over the past few years, offset by a rapid growth of digital clients and a skyrocketing number of online transactions. At the same time, more traditional customers continue to value physical presence and face-to-face interactions. This bifurcation of customer needs is a challenge all banks face. Creating an omnichannel environment that caters for the increasingly diverse client base is a huge undertaking, but it can be expedited using intelligent tools, while also leading to significant returns on investment.
Working out an appropriate AI-based innovation strategy that solves real problems and adds value is no small feat. Get it right and, as our experience indicates, you will see a rise in engagement, product use, and profitability. But getting it right requires deep data centralisation, creation of channels tailored to different client needs, and a joined-up smart platform underpinning them. Sticking an AI label is no longer enough for the digitally savvy client – significant groundwork is required for AI implementations to be a true success and help retain clients over the long term.
By Cristina Lázaro, head of CaixaBank Business Intelligence