Liquidity risk COVID-19: big vs small data
Using the right technologies to track, trace, and manage liquidity data and reporting will help financial institutions smoothly navigate the balance sheet and financial liquidity issues arising from the pandemic.
The Turing Machine phenomenon
During World War II, leaders of the free world called on industries to get creative and focus on critical warcraft needs. A technology boom ensued. The Turing Machine – with which the allies cracked the Enigma code – was one of many tech miracles of the era that turned the course of the war. Effectively the first risk engine, the machine enabled users to analyse (decrypt) critical data, understand (enemy) positions, and thus mitigate risks.
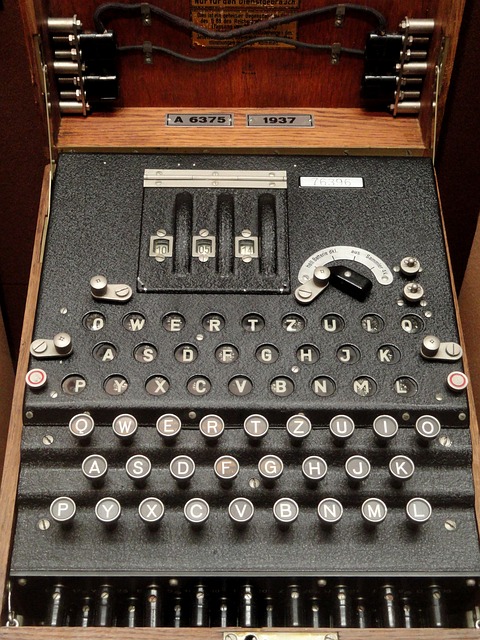
Banks must be prepared to perform deep due diligence on their credit lines and loan books.
In today’s war against coronavirus (COVID-19), industries are also answering the call to invent, retool, and ramp up to deliver vital medicines, specialised equipment, and supplies. To mitigate and beat the coronavirus, there has already been an explosion of new capabilities that capture, map, and enable users to understand COVID-19 infection data. Just as before, we can expect this global crisis to continue to precipitate technology changes into the next decade.
A liquidity pandemic and a changing regulatory landscape
As financial institutions navigate COVID-19’s devastating economic impacts, managing liquidity risk and maintaining regulatory compliance is more challenging than ever. Organizations seek new technologies that can help them track, trace, and manage their risk and regulatory data and better understand the crisis’ impact on the balance sheet and financial liquidity.
Under Basel standards, banks must calculate their daily liquidity coverage ratio (LCR). And a major change now facing banks is around the new liquidity stress testing (LST) requirements. Behind the new requirements is a big data ask: more data, reported more frequently. Especially for the global systemically important banks (G-SIBs) that have the largest liquidity thresholds and must manage the largest liquidity positions, this is a huge data ask.
To comply, financial institutions may have to adopt new models, create new scenarios, and run them concurrently. Regions may implement the Basel-drivers differently, but the challenges financial institutions face on the liquidity front and from the big data requirement are similar.
While the new Basel IV standards have been widely accepted In Asia-Pacific, plans for their adoption differ across the region. And the types of deployment under consideration and deemed acceptable also differ. Cloud deployment is a case in point. Whereas the Australia Prudential Regulatory Authority (APRA) for example, has no issue with banks’ data being hosted in the cloud for regulatory purposes, Indonesian regulators insist that banks’ data be resident within their borders.
For financial institutions with reach spanning across many jurisdictions in Asia-Pacific, these regional differences in Basel adoption plans and deployment options create many logistic data challenges. To manage liquidity reporting for a consolidated group without being able to track data in one format on a single platform, requires excessive intervention and resources.
And financial institutions are aware that other new regulations will also require large-scale data management and sophisticated calculation capabilities. A good example is the pandemic-postponed inception in January 2023 of the additional Basel IV regulation of the fundamental review of the trading book (FRTB) that will require banks run their internal models approach (IMA) risk calculations at a trading-desk level and adjust their data sourcing and calculation strategies accordingly.
In addition, with the economic impact of the pandemic continuing through year end, corporations and small and medium enterprises (SMEs) in Asia-Pacific most probably will need to extend their bank credit lines to obtain additional liquidity. Due to the low interest rates prevailing since 2008, its highly likely that many companies are already highly leveraged.
Therefore, banks must be prepared to perform deep due diligence on their credit lines and loan books. That exposure data must also be granular, auditable, and traceable, to withstand a degree of scrutiny perhaps never seen before.
As they manage to a panoply of COVID-19 disruptions and changing regulatory requirements, financial institutions in all jurisdictions must be able to evaluate regulatory data management and reporting technology from both ‘big’ and ‘small’ data perspectives. And, whatever technologies they deploy must not only be fast and nimble, but also transparent and auditable.
Big data vs small
Given the growing and enormous scope of the pandemic-driven liquidity data ask, big data technologies certainly are attractive. Performance enhancement applications such as Hadoop, Spark, and RedShift can help in terms of running large amounts of data.
Even more urgent, however, is that banks need to be able to track, trace, and extract data at an extremely granular level to better manage their balance sheets and the evolving liquidity landscape. Without transparent access to the ‘small’ data and being able to understand it well, enhanced speed delivered by big data technologies is redundant. Therefore, managing underlying risk and regulatory data at that ‘small’ level is of paramount importance. Eliminating the need for manual adjustments is just as critical as achieving desired performance acceleration.
Thinking small… is big
So, to fight this liquidity pandemic, thinking small is really the biggest weapon in the arsenal. Small data can be defined as the slippage that occurs when a bank uses a risk engine to calculate data emanating from multiple data sources. Most of the time, most of these multiple sources present incoherent and missing data that requires extra monitoring and special preprocessing.
To cope with slippage, financial institutions usually have to make numerous manual adjustments and have to allocate additional, costly dedicated resources to executing the process. Clearly, financial firms can build or buy a risk engine that can offer functionalities such as value-at-risk (VaR) calculation, risk reporting, and stress testing. However, if the organisation’s small data is not all present and correct in terms of lineage, then the calculation engine is redundant.