Why the focus on risk weighted asset optimisation is increasing
The ongoing pandemic continues to impact banks and financial institutions globally. Regulatory bodies have reduced interest rates and provided moratoriums to ease the financial pressures on the end consumers, but the impact of such moves have resulted in a severe liquidity crunch for the financial institutions thereby impairing their profitability.
To reduce the liquidity pressure on the industry, regulators and central banks have also taken certain steps such as:
- The US Federal Reserve purchasing $700 billion of longer-dated bonds to help markets function smoothly,
- Bank of England putting its stress test on hold this year and advising banks to tap into £23 billion from their countercyclical capital buffers, reducing the same to 0% for next 12 months,
- In Europe, EU-wide stress test exercises have been postponed to 2021.
While the above directives have provided some relief to banks these institutions are also assessing alternative avenues to help them free-up capital that can enable them to seamlessly continue with business operations. One such opportunity lies in optimising any risk weighted assets (RWAs), which is an integral part of the capital adequacy ratio (CAR) calculation process.
The capital adequacy ratio is a measure of a banks’ core capital expressed as a percentage of its RWA and the final number should be greater than 8 %. These calculations are governed by the Basel Accord which is published by Banks for International Settlement (BIS) and further enforced by national regulators in their respective geographies. National regulators track a bank’s CAR to ensure that it can absorb a reasonable amount of loss and complies with statutory capital requirements.
The process of RWA calculations has existed for quite some time within banking and has undergone multiple iterations from Basel I to Basel III, with Basel IV on the anvil of adoption. The entire process of RWA calculation touches across multiple areas like data management, modelling and reporting. In many banks, the entire process is not seamless and is often saddled with challenges which eventually results in higher RWAs. Since the RWA computation process has a direct impact on the amount of capital held by a bank, an improper process may result in higher RWA numbers resulting in a liquidity squeeze.
The below diagram illustrates some of the key contributors for higher RWAs in banks.
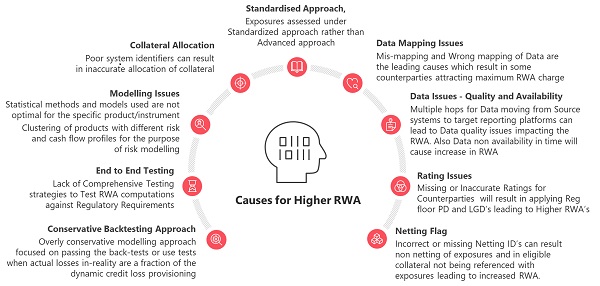
Source: TCS
The current pandemic has also brought in a liquidity squeeze for banks globally and banks are turning to newer avenues to ensure they have adequate capital available for business operations. While the concept of RWA optimisation has existed for some time, the existing situation has bought the initiative more in focus as a capital optimisation initiatives and banks are looking to mitigate the aforementioned challenges to improve their capital provisioning.
The creation of a dedicated RWA optimisation team can assist banks with these challenges. This team will be placed in between the business and IT teams and will analyse the current RWA processes and list out key issues in the current process from a data, systems, computation, modelling and reporting perspective.
This team can make a detailed assessment of all the issues and their capital impact on the current RWA numbers. The RWA optimisation team, in consultation with the business and IT teams, will need to then formulate a remediation plan to address the issues and subsequently create a plan of action to implement and monitor the changes.
An indicative high-level approach for RWA optimisation is illustrated in the figure below.
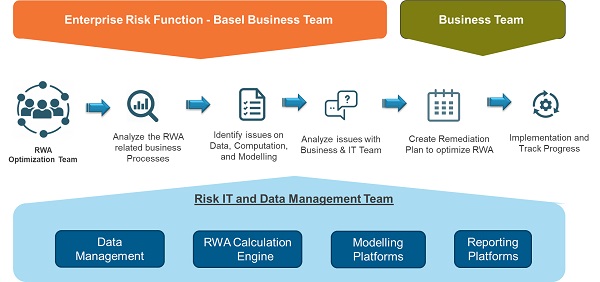
Source: TCS
Some banks have kickstarted these initiatives more so after the pandemic and have been able to show some savings from this initiative by changing business processes, reviewing data issues and evaluating their existing models.
As the concept is gaining more traction in the industry, many banks have started formulating an action plan and framework to drive this initiative within their organisation. Some are even leveraging digital technologies like machine learning, robotic process automation, artificial intelligence etc, to gain the required edge in driving this initiative.