Teaching AI to have a heart
Financial services companies increasingly rely on AI to make decisions that humans used to make, creating efficiencies for the companies and lowering costs.
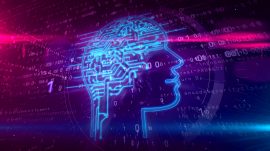
Unpicking bias in AI is an opportunity for fintech and one that is very needed
Where these decisions are customer-related, these customers are now at the mercy of algorithms. In theory, this should be a good thing.
Algorithms don’t feel emotions and therefore make decisions based on hard facts and data points, which means that human traits of conscious and unconscious bias should not feature. And yet, it appears that AIs have become an extension of the humans that have programmed them and carried their biases through.
I recently read a fascinating article in Time about Uber’s problems with AI.
Uber uses AI-driven facial recognition to verify drivers. However, some drivers say they found themselves locked out of the Uber app because the AI deemed them to be fraudulently trying to access it.
According to the drivers and trade union the Independent Workers’ Union of Great Britain (IWGB), the problem seemed to be that the facial recognition technology had trouble with darker skin tones.
In a recent conversation with Kareem Saleh from a start-up called Fairplay, I was confronted by the harsh realities of AI-driven bias in the financial services sector.
Kareem showed me a series of infographics illustrating lending decisions made for home loans in the US. The data source is the lenders themselves, legislated to collect and report ethnicity and gender as part of the process.
Fairplay has collated all the available data and uses it to power a dashboard that shows down to county level lending decisions. The data shows a shocking bias based on ethnicity and gender. The negative bias is particularly acute for Black people, although Hispanic and Native Americans do not fare much better. Women are also more likely to be disadvantaged than men.
Seeing the comparisons is sobering.
Results can be shown by institution, and for most, I would say that it makes incredibly uncomfortable viewing. Black people in many areas are 80% less likely to get a positive outcome.
When Kareem first showed me the infographics, I had assumed (perhaps naively) that the results were based on a human-driven process. So, it was all the more shocking to discover that the results were driven by machines.
I asked Kareem what the best approach was to solve the problem. He responded that “the first thing to do is a diagnosis”. Kareem told me that Fairplay has an analysis tool that analyses a bank’s existing lending software for signs of discrimination. It tries to answer the following questions:
- Is the algorithm fair?
- If not, why not?
- How could it be fairer?
- What’s the economic impact to the business of being fair?
- Do applicants who are rejected get a second look to see if they might resemble favoured borrowers?
Answering these questions forces institutions to look at their decision engines and find ways to re-train them.
Re-evaluating declined loan applications happens using more complete information about the borrowers and different modelling techniques to see if they resemble creditworthy people. So, for example, women tend to have inconsistent employment between 25 and 45. This would be a creditworthiness flag for male borrowers but not necessarily for women taking career breaks to raise children.
Kareem knows that lenders will increase their approval rates for female, Black, and other non-White people by re-training algorithms and taking a second look at rejected customers, particularly those just below the approval threshold. By his estimates, this increase can be 10-30%, which is huge.
The danger for all of us is that AI becomes a blunt instrument, making decisions based on the data that it is being given rather than looking more broadly.
As machines make more decisions, consumers will want to know that those decisions are made fairly. It is not just consumers either. Regulators are prioritising diversity and inclusion. They can see removing bias and increasing fairness will benefit the sector.
Frankly, we who work in the industry should be doing all we can to ensure that race and gender have little influence on machines’ decisions.
Unpicking bias in AI is a whole new fintech opportunity and one that appears to be very needed.
So, if I were an institution, I would be looking carefully at my algorithms and AI, asking Kareem’s five incredibly sensible questions, and then doing something about it!
About the author
Dave Wallace is a user experience and marketing professional who has spent the last 25 years helping financial services companies design, launch and evolve digital customer experiences.
He is a passionate customer advocate and champion and a successful entrepreneur.
Follow him on Twitter at @davejvwallace and connect with him on LinkedIn.