How can neobanks meet the challenges presented by cybercrime?
With the number of digital-only ‘neobanks’ in the UK on the rise, the top four alone have over 12 million account holders between them, according to research by Insider Intelligence.
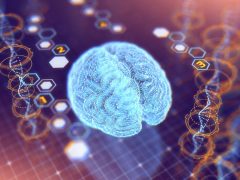
Advances in AI and ML could help neobanks better protect themselves against cybercrime
As a rapidly expanding niche, they represent a major shift in a traditional market, driven by the ubiquity of connected devices and a younger generation of customers who gravitate towards digitally-native services.
Operating without physical branch networks and complex legacy technology infrastructure, neobanks are considered by many to be the future of the industry. And while their emergence has done much to challenge the status quo, promote innovation and competition, they operate within a financial monitoring ecosystem that is still based on the assumption of a human-centric relationship between bank and customer.
With neobanks’ customer relationships defined by apps and APIs, this creates a particular set of challenges at a time when financial crime is becoming increasingly sophisticated and successful.
If that wasn’t enough, the integration of digital technologies and criminal strategies that exploit cybersecurity vulnerabilities is threatening to elevate issues such as fraud to a scale not seen before. The desire to acquire as many clients as possible via frictionless onboarding processes can often leave insufficient time for rigorous financial crime prevention methods.
Indeed, financial and cybercrime are becoming increasingly unified, as criminals look to exploit cybersecurity vulnerabilities to focus on everything from document and identity fraud to account takeovers and card crime.
This contributes to a major financial burden on consumers and businesses alike. For instance, a recent study from Juniper Research indicates that between 2021-2025, online payment fraud will cost in excess of $206 billion.
Complex problems need innovative solutions
While neobanks have focused on innovation in an effort to differentiate themselves from their ‘high street’ rivals, what hasn’t changed is the approach they take to financial fraud, which tends to follow the same processes and rules as their long-established counterparts. Often those processes may be under-resourced as neobanks strive to be as lean as possible to prioritise growth.
Digital innovation is also improving the effectiveness of financial criminals who are turning to automation techniques to increase the reach and volume of their attacks. This puts immense pressure on legacy detection and prevention systems and processes.
The problem is, identifying and defeating fraud remains largely reliant on human-centric, labour-intensive processes, even in neobanks. Teams are often overworked with the result that their finite resources are stretched across too few cases. Add to that the major burden placed on fraud teams by the huge levels of false positives – where legitimate transactions are incorrectly flagged as suspicious – and defences are arguably under greater pressure than ever.
The knock-on effect is that neobanks are using valuable resources to manually tackle fraud when instead, they could allocate the time, expertise and creativity of their people to pushing the boundaries of banking innovation further still. And this isn’t just a question of bottom-line impact. There is also the human cost of finance professionals facing burnout and organisations dealing with the accompanying staff turnover that has the scope to further degrade performance.
Faced with this scenario, how can neobanks or any financial institution keep pace with automated cybercrime unless they can employ similar techniques to meet the challenge head-on?
Artificial intelligence (AI) and machine learning (ML) powered technologies enable financial organisations to scale prevention and detection to a level manual processes can’t hope to match. It can, for instance, be applied when authenticating genuine documents so teams can focus their valuable time addressing more sophisticated document fraud.
In anti-money laundering (AML) use cases, AI can also be applied to analyse the hidden relationships between identities and transactions to draw a better decision boundary between legitimate and criminal activities. And for buy now, pay later (BNPL) and payment fraud, it can be applied to accurately detect real vs stolen identities, reduce the frequency and impact of account takeovers and minimise the business burden of false declines.
The list of use cases is growing all the time. Not only do these technologies improve productivity, but they also boost detection rates while driving down losses. What’s more, they help deter fraudsters who must put more time and resources into their efforts for a less favourable return.
Without innovation-led change driven by advances in AI and ML, neobanks could find themselves severely impacted by rising levels of fraud. At a time when scrutiny and compliance are significantly increasing, with several high-profile organisations being fined in recent times, meeting the challenges presented by cybercrime will help them focus on turning predictions of industry transformation into reality.