ChatGPT and generative AI – what does banking have to do with it?
Not a day goes by that we don’t hear something about generative artificial intelligence (AI).
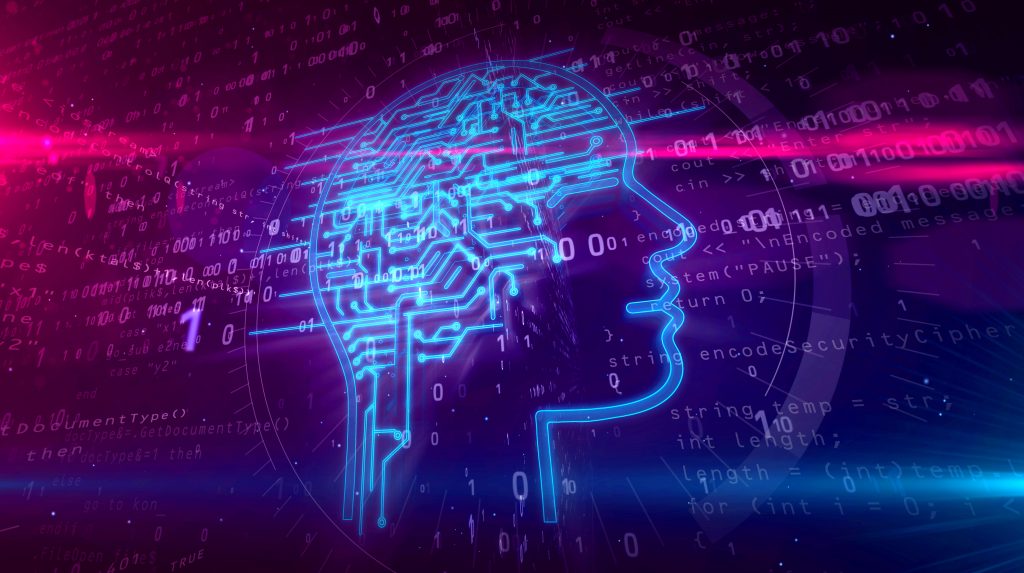
Ready or not, the generative AI genie is out of the bottle and there is no going back
Ready or not, the genie is out of the bottle and there is no going back. From digital art and journalism to gaming and marketing tech, every industry is being disrupted.
Open AI’s ChatGPT, in particular, stole the limelight after it was revealed that CNET has been experimenting with the tech and publishing articles – some reportedly riddled with errors – written by the bot for months.
While generative AI tools might seem to be able to generate human-like responses, there is often no transparency as to how the AI is trained and where the data comes from.
Accuracy (or lack thereof) presents another layer of challenge when the answers are not cited nor fact-checked by humans. We saw an interesting example recently when ChatGPT was trying to explain why the size of a cow egg is different than that of a chicken egg – even though cow eggs don’t exist to begin with.
While users can view the source of a search and decide for themselves what to trust, there is no way of proving the legitimacy of the results from an AI-powered chatbot search. So even though on one hand it may be amusing to think that a bot treats a “cow egg” as real, it could have catastrophic results if the bot provides seemingly authoritative-sounding medical or financial advice that is just plain wrong.
It is no wonder that some financial institutions are reluctant to experiment with the technology for now. Others such as Citi Group, Bank of America, Deutsche Bank, Goldman Sachs, and Wells Fargo have outright banned it. While some might be tempted to “move fast and break things”, this is probably not the best course of action. For an industry where trust is paramount, it is even more crucial for us to foster a customer-centric experience based on transparency and trust. Without understanding what is in the dataset that is used to train the tools, how can we be certain that we are not perpetuating the biases in the input data and causing society more harm than good?
Imagine a scenario where a bank leverages a generative AI tool to conduct research for a potential investment. The tool could potentially create fictional references that do not exist and may also plagiarise someone else’s work using the data that it crawls from the internet. Not to mention the insights and recommendations may not even be correct to begin with.
For businesses that thrive on maintaining personal relationships with customers in return for lucrative returns, it would seem rather impersonal to simply repurpose strings of text that can otherwise be obtained freely with a web-crawling bot.
So, where do we go from here?
I have written previously about the need to embrace slowness and hit reset — on both a personal level as well as a professional level. The same applies to innovation where there is so much at stake. We should aim to avoid scenarios where we can easily repeat if not amplify mistakes from the past.
In what has been unfortunately termed the “AI arms race”, what we need more of is not speed; rather, it is genuine dialogue on how to roll out the technology in a more thoughtful and responsible way. Or else, this will just be a race to the bottom, at a cost that we can ill afford.
About the author
Theodora (Theo) Lau is the founder of Unconventional Ventures. She is the co-author of Beyond Good and co-host of One Vision, a podcast on fintech and innovation.
She is also a regular contributor for top industry events and publications, including Harvard Business Review and Nikkei Asian Review.