Machine learning vs human experience in invoice finance
Machine learning is taking over more previously manual human tasks across all industries and the financial services sector is no exception. In fact, fintech is driving rapid change across the whole sector including invoice finance.
The use of incredibly clever computer algorithms which can be programmed to learn as new data comes in is and will undoubtedly result in huge improvements in certain areas of invoice finance provision.
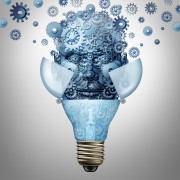
Fintech is driving rapid change across the whole sector.
However, human experience and judgement will remain a vital part of the process as even the most complex machines have their limitations.
Machine learning is a subset of artificial intelligence by which computer systems are developed that can automatically learn and improve from experience and new data without having to be reprogrammed or instructed. Widely used for complex data analysis, machine learning can quickly process huge amounts of information and spot trends and patterns invisible to humans.
There are some key areas where emerging technology is being applied to change the way we operate introducing greater efficiency and productivity which benefit end user businesses.
Credit checking and lending decisions
Traditionally credit checks and lending decisions have relied upon an experienced staff member to pull together and analyse all the relevant background information on a company, carrying out due diligence in as much detail as possible. Having done this, they would use their knowledge and experience to make an informed decision about whether to lend or not and what conditions to impose.
The data input required to carry out comprehensive credit and background checks on a company can be huge. Each client and potential client has dozens of their own customers which raise multiple invoices as well as many other factors that must be assessed. The man hours and mathematical know-how required to process, check through and accurately analyse this amount of data is huge.
In truth, even the most experienced of analysts will fall victim to human error, unable to spot irregularities and patterns that a well programmed computer system can see. So, machine learning technology is facilitating much quicker and more accurate checks on companies to ensure any potential hiccups or defaulters are spotted ahead of time.
This benefits everyone as lending decisions can be made quicker and lenders can offer better terms in greater confidence that their debtors have undergone rigorous checks.
However, machines have their limitations. They’re only as clever as the data they have been trained on. As inevitable changes to the way businesses operate occur from time to time, driven by law, innovation and other external factors, these intelligent systems can struggle to keep pace.
Ultimate decisions in invoice finance should still rest with a seasoned professional and not a machine. Machine learning systems should be viewed as an incredibly powerful tool rather than a robotic staff member. So, where company checks throw up concerns, the machine can alert staff who will then look at it in more detail. This is how machine learning is best used – as an aid to staff not a replacement!
There are now several AI-powered invoice financing platforms but I would be wary of a lender with no human face as there is always the odd previously unforeseen complication which no computer programme, however clever, will be prepared for.
From the customer perspective, a machine learning programme will not offer the same added value client service that a good invoice financier should provide. We pride ourselves in being trusted advisors to many of our customers sharing knowledge and insight on all aspects of running a business, far beyond purely paying the value of their invoices.
Fraud detection
Fraud is a growing problem as virtually all business deals have become faceless online transactions. Detecting fraud is often very difficult, requiring the ability to process and analyse huge reams of data to flag anything unusual or irregular. This is simply not realistic or possible for staff in most companies and is an area where machine learning has proven most effective.
Machine learning technology can access neural networks of data and filter through masses of information and transactions to spot very early on if a company is behaving in a way that should spark concern.
There have been cases in the industry where fake debtor companies have been set up and registered with Companies House with named directors who appear to have clean records. These companies then create fake invoices and submit them to the client company. In these cases it can be very difficult to get any redress.
Machine learning can also prevent companies that are in financial difficulties borrowing more than they can safely pay back, again by flagging it early when patterns of behaviour alter. Desperate directors will sometimes resort to tricks such as factoring their invoices and claiming monies provided by their invoice financier while also demanding the payment from their customers at the same time, thus getting a double injection of cash. While this might plug a cash shortfall short term it will leave the company owing even more money further down the line so it’s not a sensible solution – and will go against any legal agreement with the finance provider rendering the directors liable to prosecution.
The key thing to remember about machine learning is that its analytical abilities will only ever be as good as the data input so large amounts of relevant information are key to successful machine learning outcomes. Companies with access to limited data would feel far less benefit, collaboration within financial services and access to industry wide data is essential to weaponise AI in the fight against fraud.
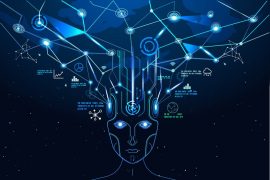
Be wary of a lender with no human face as there is always the odd unforeseen complication.
The future
Technology is advancing all the time and can now analyse information far more complex than a set of figures and carry out increasingly nuanced human tasks such as reading contracts.
We will soon see many more manual tasks moved over to machines with humans handling only the more complex cases and tasks requiring a more personal approach such as elements of client communications.
An example of where the machine and human can work really well in partnership is in sales. A machine learning programme can be set a task to analyse a specific industry sector and flag all the prospects worth approaching for new business. An experienced salesperson can then be provided with the refined list created by the programme to start making calls and drafting emails. As technology moves forward, it’s feasible an AI salesperson will handle the initial communication and only involve a human at the latest possible stage.
This steadily advancing automation is unlikely to mean staff are sitting idle but will simply result in companies becoming far more efficient and getting much more work done.
By James Beck, CTO of Optimum Finance