AI rewrites 21st century debt collection practices
Artificial Intelligence (AI) technologies are the focus of intense excitement at the moment, and nowhere more so than in the fintech sector. In just the last year, we’ve looked at the rise of AI in retail banking, and also explored whether AI is the future of commercial lending. And while many of the promises of AI have yet to be realised, it’s increasingly apparent that everyone is looking to the technology to revolutionise the banking industry.
The same cannot be said for debt collection firms. To say that these firms have an image problem is to understate the negativity with which they are regarded by both consumers and a new breed of responsible fintech companies. Think of debt collection, and the immediate image that comes to mind is a string of threateningly incessant phone calls demanding money from scared debtors. Forward-thinking creditors have begun to wonder if such tactics are efficient or effective.
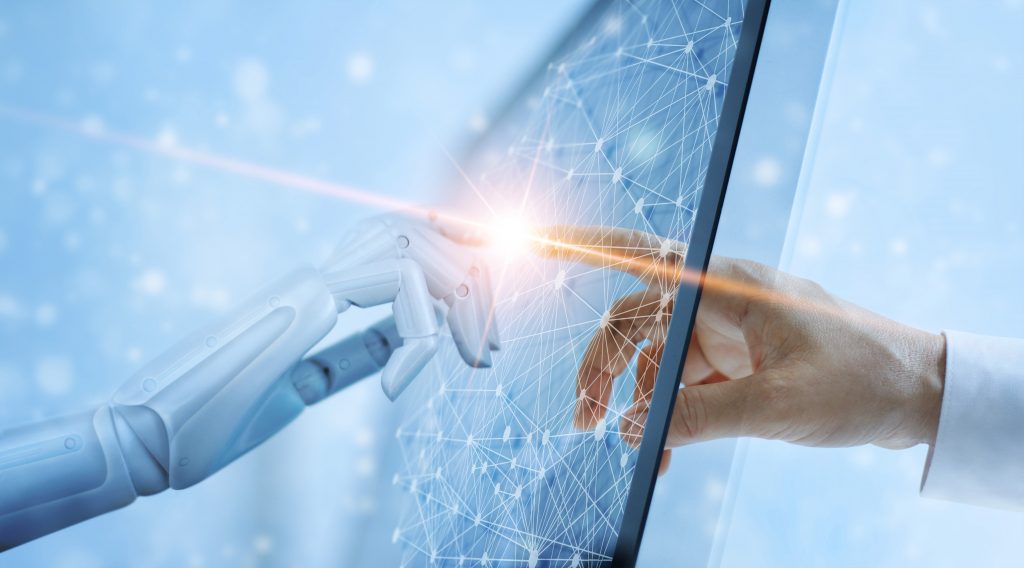
It’s likely that AI will find a range of uses in the debt collection sector.
It doesn’t have to be like this, some debt collectors have realised, and are looking to change stereotypes via innovative uses of technology. AI is a key component of this. In this article, we’ll look at how they are doing so.
Data fragmentation
First, it helps to understand the challenges faced by debtors and debt collection agencies in the effective, quick resolution of debt claims. Contrary to the popular image of debt collection, a significant number of bills go unpaid because of missed communications, rather than due to customers not having funds available. A debtor moving can trigger a cascade of missed correspondence and escalating costs, leaving them unable to pay their debt by the time they become aware of it.
This kind of data fragmentation is familiar to network engineers, but continues to create real problems for debt collection agencies and debtors alike. One of the major issues is that debtors, on being contacted by collection agencies, will ask for a copy of the original invoice. By law, collection agencies must supply this to prove the debt is valid. Unfortunately, what should be a simple matter turns chaotic since it often involves navigating various diverse client IT systems.
Chatbots and security
This kind of data fragmentation is an issue that AI systems could solve. One of the primary uses of this technology within financial organisations is to sift through massive collections of documents, and tag these with labels relating to the case they are part of. Deploying AI systems “behind the scenes” in this way, to look through and sort documents relating to debt claims, would almost certainly contribute to a more timely process all the way around.
Another is the deployment of AI chatbots that can be trained to effectively deal with customer queries. Though chatbots of this type are commonly used in other sectors – and even in other parts of the financial sector – using them in debt collection agencies presents particular difficulties.
This is partially the consequence of the kind of data fragmentation we’ve already mentioned: when a customer gets in touch with a debt collection agency, the first step is typically to undertake a complex and sensitive authentication procedure. With the spectre of online security threats higher than ever, from both freelance hackers and organised international government conglomerates, it is incumbent upon a debt collector to verify beyond doubt they are, in fact, speaking to the actual person on the account.
AI could, in theory, help with this authentication process. Systems are being developed that can use a customer’s voice as part of identity checking processes. While there remains a danger of this kind of biometric databases being hacked and sensitive financial information becoming public knowledge, AI algorithms and machine learning techniques offer a real chance at achieving better security.
Understanding debt
The uses of AI we’ve already mentioned are aimed primarily at improving communication between debt collection agencies and customers. However, there is another, broader way in which AIs can be used to improve the efficiency of the debt collection sector: by furthering our understanding of consumer debt itself.
One of the major advantages of AI systems is that they are able to understand vast amounts of data faster than a human operator, and for customers with complex financial histories and equally complex needs, this could mean that AIs are better at designing repayment plans than their human colleagues.
Using AIs in this way will be challenging, however. Given the fragmentation of data we’ve mentioned, it’s difficult to imagine that AIs will have access to all the relevant facts to be able to propose a legally binding repayment plan. And in Europe, such automated decision making is explicitly prohibited by Article 22 of the GDPR.
Perhaps, a broader approach is needed. Some of the most exciting uses of AI in the past decade have been those that seek to use the pattern recognition abilities of these systems to analyse vast data sets, and reveal patterns of human behaviour that were previously invisible. As report after report has noted, consumer debt is a major problem in the world’s advanced economies but understanding its causes lags far behind our knowledge of its consequences.
Responsibility and profit
Ultimately, it’s likely that AI will find a range of uses in the debt collection sector. One of the clearest – and most pressing – applications will be to allow information and documents on individual cases to be collected together from multiple systems and made available for the people who need them.
However, this is only the beginning. If AIs can provide an insight into why people get into debt in the first place, and how they can get out more effectively, then it might be that AI can make lending more responsible. And that’s good news for both debtors and debt collection agencies.